interpolation, differentiation에 이어서 integration을 수치해석적으로 수행해보겠습니다.
1. Introduction
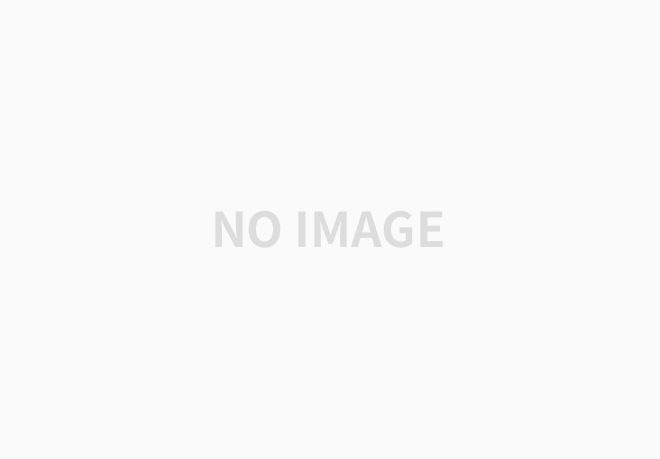
x0(=a)x0(=a)부터 xn(=b)xn(=b)까지 적분을 하려고 합니다.
우리는 discrete하게밖에 계산할 수 없으므로,
I=∫baf(x)dx=∑nj=0fj⋅ωjI=∫baf(x)dx=∑nj=0fj⋅ωj
적분을 오른쪽 식처럼 계산해야합니다. 이 때 ωjωj는 weighting factor라고 부릅니다.
이 전에 Lagrange polynomial을 배웠고, 그 polynomial 식은 p(x)=∑nj=0fjLj(x)p(x)=∑nj=0fjLj(x)입니다.
이 방법을 통해 내가 알고 있는 데이터 점들을 충족하는 함수 식을 구할 수 있습니다.
Lagrange polynomial로 적분을 수행한다면
I=∫bap(x)dx=∫ba∑nj=0fjLj(x)dx=∑nj=0fj∫baLj(x)dxI=∫bap(x)dx=∫ba∑nj=0fjLj(x)dx=∑nj=0fj∫baLj(x)dx
여기서 ∫baLj(x)dx∫baLj(x)dx이 weighting factor처럼 작용합니다.
Cotes number를 도입하겠습니다.
Cotes number : Cnj=1b−a∫baLj(x)dxCotes number : Cnj=1b−a∫baLj(x)dx
Newton-Cotes formula : I=(b−a)∑nj=0fjCnj(x)Newton-Cotes formula : I=(b−a)∑nj=0fjCnj(x)
후에 배울 numerical integration method는 Newton-Cotes formula의 variation입니다.
예를 들면,
if f=1 then ∑nj=0Cnj=1if f=1 then ∑nj=0Cnj=1
n=1n=1이라고 할 때 x0=a,x1=bx0=a,x1=b입니다.
L0(x)=x−ba−bL0(x)=x−ba−b, L1(x)=x−ab−aL1(x)=x−ab−a
C10=1b−a∫bax−ba−bdx=12C10=1b−a∫bax−ba−bdx=12
C11=1b−a∫bax−ab−adx=12C11=1b−a∫bax−ab−adx=12
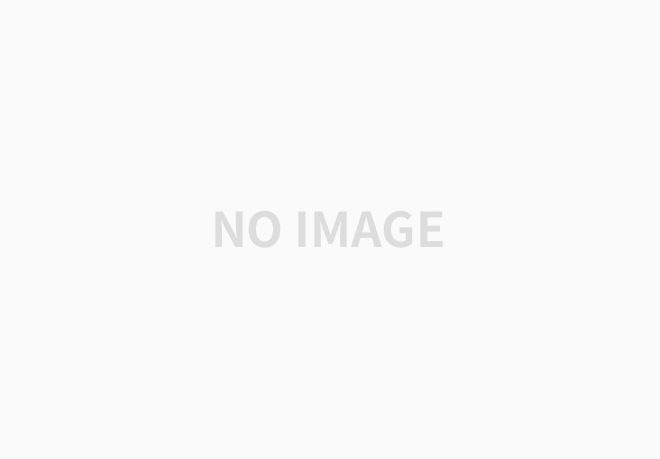
I=(b−a)[f0C10+f1C11]=b−a2[f0+f1]I=(b−a)[f0C10+f1C11]=b−a2[f0+f1]
이 방법은 후에 배울 Trapezoidal method에 대한 식입니다. 이에 대한 order of accuracy θ(h2)θ(h2)
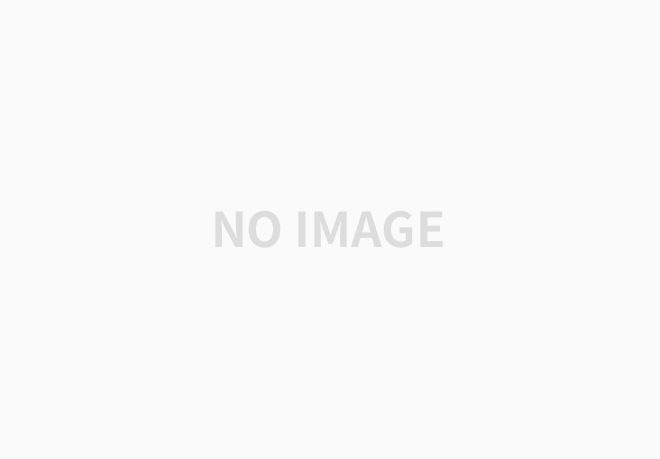
n=2n=2인 경우에 대해서 똑같이 수행하면 ∫baf(x)dx=(b−a)6[f(a)+4f(a+b2)+f(b)]∫baf(x)dx=(b−a)6[f(a)+4f(a+b2)+f(b)]
이 방법은 simpson's rule이라고 합니다. θ(h3) θ(h3)
이 때, n이 늘린다고 해서 항상 좋은 것은 아닙니다.
2. Rectangular (mid point) rule
Numerical integration 방법 중 하나인 mid point rule에 대해서 설명하겠습니다.
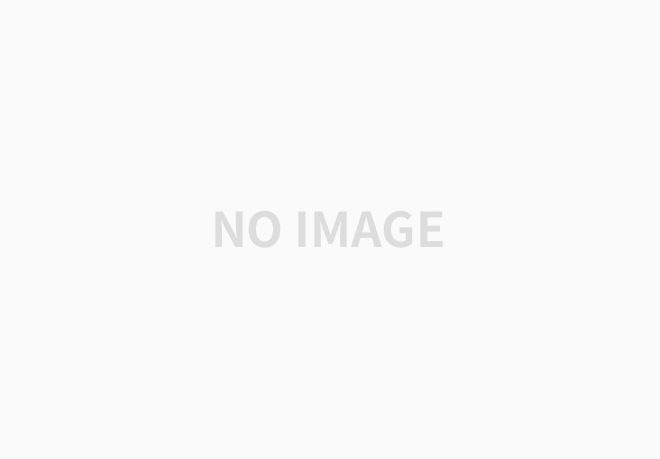
mid point rule은 이름에서 알 수 있는 것처럼 두 점 사이를 적분하고자 할 때 중앙값을 사용하는 방법입니다.
∫xi+1xif(x)dx≅hif(yi)+θ(hpi)⋯(∗)∫xi+1xif(x)dx≅hif(yi)+θ(hpi)⋯(∗)
hi=xi+1−xihi=xi+1−xi
Taylor series expansion을 이용해서 f(x)f(x)를 yiyi에 대해서 표현하겠습니다.
f(x)=f(yi)+(x−yi)f′(yi)+(x−yi)22!f″(yi)+(x−yi)33!f‴(yi)+⋯
이 식을 (∗) 좌변에 있는 f(x)에 대입한 다음에 적분을 수행합니다.
그 결과,
f(yi)hi+12(x−yi)2f′(yi)|xi+1xi+13(x−yi)32!f″(yi)|xi+1xi+⋯
(x−yi)2 등의 짝수 제곱 항은 0이 되고 홀수 번째 항만 살아남습니다.
∫xi+1xif(x)dx≅hif(yi)+θ(hpi)=hif(yi)+124h3if‴(yi)+θ(h5i)
12(x−yi)2f′(yi)|xi+1xi=0 등 짝수 항은 0이 됩니다.
따라서 위 식에서 가장 큰 error term은 124h3if″(yi)이므로, Leading order of error는 3rd order가 됩니다.
3. Trapezoidal rule
trapezoidal은 사다리꼴이라는 뜻입니다.
실제로도 이 적분 방법은 두 점 사이 적분을 사다리꼴 계산으로 수행합니다.
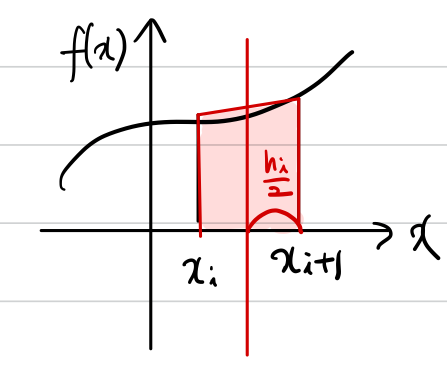
∫xi+1xif(x)dx≅hi12[f(xi)+f(xi+1)]⋯(∗)
이 trapezoidal rule과 mid point rule의 오차를 비교해보고 싶기 때문에 f(xi),f(xi+1)를 mid point인 yi에 taylor series expansion으로 표현합니다.
f(xi)=f(yi)−hi2f′(yi)+h2i8f″(yi)−h3i48f‴(yi)+⋯
f(xi+1)=f(yi)+hi2f′(yi)+h2i8f″(yi)+h3i48f‴(yi)+⋯
이 두 식을 (∗)에 대입하면,
hi2[f(xi)+f(xi+1)]=hi2(2f(yi)+h2i4f″(yi)+⋯)
=hif(yi)+h3i8f″(yi)+⋯
여기서도 짝수항은 부호가 다르기 때문에 더하면 사라집니다.
위에서 mid point rule일 때
∫xi+1xif(x)dx=hif(yi)+124h3if‴(yi)+θ(h5i)라는 것을 알고 있습니다.
trapezoidal에서는
hif(yi)=hi2[f(xi)+f(xi+1)]−h3i8f″(yi)+⋯
이기 때문에 mid point rule 식에 대입합니다.
∫xi+1xif(x)dx=hi2[f(xi)+f(xi+1)]−18h3if″(yi)+124h3if″(yi)+θ(h5i)
error term을 보면 −112h3if″(yi)가 LOE입니다.
locally 3rd order의 order of accuracy를 가집니다.
따라서 trapezoidal 방법은 mid point rule과 같은 order를 가지지만 크기는 mid point rule이 더 작고(124<112), 부호가 반대입니다.
크기가 mid point rule이 더 작기 때문에 좋아보이지만 실제로 계산을 할 때는, mid point에 대한 정보가 없기 때문에 xi,xi+1,xi+2 이렇게 3개의 데이터로 xi+1을 mid point로 쓰기 때문에 각 데이터 포인트 간의 간격이 hi에서 2hi로 증가하고, 따라서 error는 mid point가 더 커지게 됩니다.
4. Global accuracy
위에서 trapezoidal rule의 accuracy가 locally 3rd order라고 말했는데, 왜 locally라고 언급했는지 설명하겠습니다.
[a,b] 구간의 적분을 구할 때 전체 구간이 적분을 쪼개서 적분할 수도 있습니다.
I=∫baf(x)dx=∫x1x0f(x)dx+∫x2x1f(x)dx+⋯+∫xnxn−1f(x)dx
만약 각 적분에 대해 trapezoidal rule을 적용한다면
h2[f(x0)+f(x1)+f(x1)+⋯+f(xn−1)+f(xn)]
=h2[f(a)+2∑n−1j=1f(xj)+f(b)]−112h3∑ni=0f″(yi)
전체 적분의 오차를 알아내려면 −112h3∑ni=0f″(yi)를 구해야합니다.
# Mean value theorem
∑ni=0f″(yi)n=f″(¯x) (a≤x≤b) and n=b−ah
이 Mean value theorem
L.O.E=−h312(b−a)hf″(¯x)∼θ(h2)
하나의 panel 적분은 3rd order error이었지만
전체 panel 적분은 mean value theorem으로 2nd order error가 됩니다.
이전에 differentiation에서는 order of error는 error가 줄어드는 속도라고 언급했지만
여기서 error는 order 자체가 곧 error를 의미합니다.
5. Trapzoidal rule with end correction
이번에는 Trapzoidal rule에서 order를 높일 수 있는 방법에 대해서 알아보겠습니다.
∫xi+1xif(x)dx=hi(f(xi)+f(xi+1))−112h3if″(yi)+θ(h5i)
이 식이 위에서 구한 Trapzoidal rule인데요,
f″(yi)=f′(xi+1)−f′(xi)hi+θ(h2i) 이라는 것을 numerical differentiation에서 알고 있습니다. 이 식을 위의 식에 대입하겠습니다.
∫xi+1xif(x)dx=hi2(f(xi)+f(xi+1))−h2i12(f′(xi+1)−f′(xi)+θ(h5i))
I=∫baf(x)dx=hi2[f(a)+2∑n−1j=1f(xj)+f(b)]−h2i12[−f′(xi)+f′(xi+1)−f′(xi+1)+⋯]+∑n−1iθ(h5)
=h2[f(a)−2∑n−1j=1f(xj)+f(b)]−h212[f′(b)−f′(a)]+θ(h4)
맨 마지막에 있는 error term은 위의 4번 챕터에서 한 것처럼 mean value theorem에 의해서 차수가 하나 내려간 것입니다. 이제 저 식에서 모르는 것은 f′(b),f′(a)인데 이 경계값은 finite differentiation을 통해서 구할 수 있습니다.
2nd order error로 경계 미분값을 구하면 θ(h2)이고 (2nd order forward, backward difference 이용)
h212[f′(b)−f′(a)]에서 앞에 h2이 곱해져있으므로 미분값에 대한 error는 θ(h4)가 됩니다.
따라서 적분값(I)의 order of error는 θ(h4)이고, trapezoidal rule의 LOE(Leading order of error)가 locally 3rd order이고 globally 2nd order인 것에 비해 더 개선되었습니다.
이와 같이 다른 finite difference 기법을 이용해서 error를 개선할 수 있습니다.
이제 이후에는 simpson's rule 등에 대해서 다루겠습니다.
'수치해석 Numerical Analysis' 카테고리의 다른 글
[수치해석] Numerical integration (2) - Simpson's rule, Romberg integration, Adaptive quadrature (0) | 2021.04.04 |
---|---|
[수치해석/MATLAB] Lagrangian polynomial 구현하는 코드 (0) | 2021.04.02 |
[수치해석] Error characteristics/Modified wave number (0) | 2021.03.27 |
[수치해석] Numerical differentiation (2) (0) | 2021.03.27 |
[수치해석] Numerical differentiation (1) (0) | 2021.03.19 |